AI vs ML Explained Simply: Demystifying the Technologies Shaping Our Future!
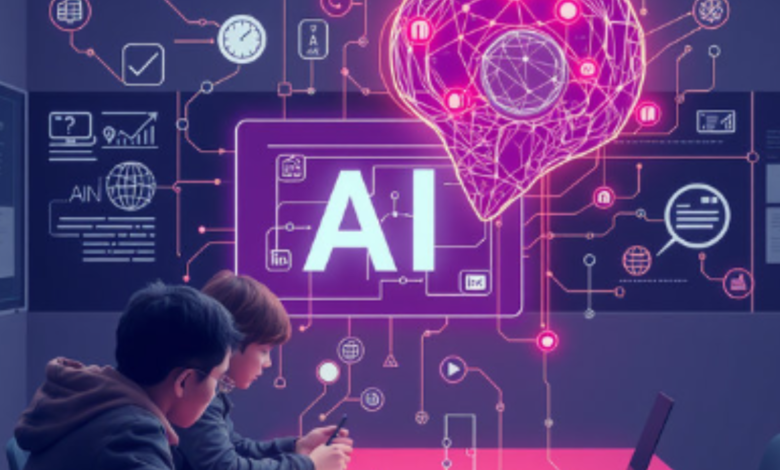
The world around us is being rapidly transformed by advancements in technology, and two of the most groundbreaking fields driving this revolution are Artificial Intelligence (AI) and Machine Learning (ML). While often used interchangeably, AI and ML are distinct concepts with unique characteristics and applications. This article will delve into the intricacies of AI vs ML explained simply, demystifying these powerful technologies and exploring there profound impact on our future.
What is Artificial Intelligence (AI) Explained Simply?
Artificial Intelligence, at its core, refers to the simulation of human intelligence in machines. It encompasses a broad spectrum of capabilities, from basic basic problem-solving and decision-making to complex tasks like natural language processing, image recognition, and even creative endeavors. The goal of AI is to create systems that can perceive their environment, reason logically, learn from experience, and ultimately, exhibit intelligent behavior.
Imagine a chess-playing computer. Traditional programming would involve meticulously defining every possible move and its corresponding response. AI, however, takes a different approach. It allows the computer to “learn” by analyzing countless games played by human experts, identifying patterns, and developing strategies that maximize its chances of winning. This learning process, while still guided by human-defined rules, enables the AI too adapt and improve its performance over time.
AI can be broadly broadly categorized into two types:
- Narrow or Weak AI: This type of AI is designed for specific tasks, such as playing chess, recommending products, or powering voice assistants like Siri or Alexa. It excels at performing predefined functions but lacks general intelligence or consciousness.
- General or Strong AI: This is the realm of science fiction, where AI possesses human-level intelligence, consciousness, and self-awareness. It can understand, learn, and apply knowledge across various domains, exhibiting common sense and reasoning abilities. WHile still largely theoretical, research in this area continues to push the boundaries of what’s possible.
The applications of AI are vast and ever-expanding. From self-driving cars and medical diagnostics too fraud detection and personalized recommendations, AI is revolutionizing countless industries and transforming our daily lives. As AI continues to evolve, we can expect even more innovative and impactful applications in the years to come.
What is Machine Learning (ML) Explained Simply?
Machine Learning, a subset of AI, focuses on enabling machines to learn from data without explicit programming. INstead of relying on predefined rules, ML algorithms are designed to identify patterns, make predictions, and improve their performance based on the data they are fed. This data can take various forms, including numerical data, text, images, and audio.
One of the key concepts in ML is the idea of training a model. This involves feeding the algorithm with a massive dataset and allowing it to learn from the information contained within. Through this process, the model identifies patterns, relationships, and trends, gradually improving its ability to make accurate predictions or decisions. For For example, a spam filter uses ML algorithms to analyze emails, identify patterns in spam messages (like suspicious senders, subject lines, and content), and learn to distinguish spam from legitimate emails.
There are are several key types of ML algorithms, including:
- Supervised Learning: In this approach, the algorithm is trained on a labeled dataset, where each data point is associated with 1 a specific outcome. FOr example, an image recognition algorithm might be trained on a dataset of images labeled as “cat” or “dog,” allowing it to learn to accurately classify new images.
- Unsupervised Learning: This type of algorithm is used to identify patterns and structures in unlabeled data. For example, customer segmentation algorithms can group customers based on their purchasing behavior, even without prior knowledge of their preferences.
- Reinforcement Learning: This approach involves training an agent to interact with an environment and learn from the consequences of its actions. For example, a self-driving car can be trained using reinforcement learning to navigate traffic by rewarding successful maneuvers and penalizing collisions.
ML is rapidly transforming various sectors, from healthcare and finance to marketing and entertainment. By analyzing vast amounts of data, ML algorithms can provide valuable insights, improve decision-making, and unlock new levels of efficiency and innovation.
AI vs ML Explained Simply: Understanding the Key Differences
While While closely related, AI and ML are distinct concepts with key differences:
- Scope: AI is a broader concept that encompasses all efforts to create intelligent machines, while ML is a specific subset of AI focused on enabling machines to learn from data.
- Approach: AI aims to simulate human intelligence in machines, while ML focuses on developing algorithms that can learn from data and make predictions.
- Focus: AI is concerned wtih achieving intelligent behavior, while ML is primarily concerned with enabling machines to learn from data.
Analogy: Imagine a chef preparing a meal. AI represents the overall goal of creating a delicious and satisfying dish. ML, on the other hand, represents the specific techniques the chef uses to achieve this goal, such as learning to cook from recipes, experimenting with different ingredients, and refining their skills over time.
AI vs ML Explained Simply: Real-World Applications
The applications of AI and ML are vast nad ever-expanding, impacting our lives in numerous ways.
- Healthcare: AI and ML are revolutionizing healthcare by enabling earlier disease detection, personalized treatment plans, and more efficient drug discovery. ML algorithms can analyze medical images, identify genetic risk factors, and predict patient patient outcomes, leading to improved patient care and reduced healthcare costs.
- Finance: AI and ML are transforming the financial industry through fraud detection, credit risk assessment, algorithmic trading, and personalized financial advice. ML algorithms can analyze vast amounts of financial data to identify anomalies, predict market trends, and provide valuable insights for investment decisions.
- Autonomous Vehicles: Self-driving cars rely heavily on AI and ML technologies, including computer vision, sensor fusion, and decision-making algorithms. ML algorithms enable these vehicles to perceive they’re environment, navigate traffic, and make real-time decisions, paving the way for safer and more efficient transportation.
- Customer Service: Chatbots and virtual assistants powered by AI and ML are transforming customer service by providing instant support, answering frequently asked questions, and resolving customer issues efficiently. These technologies can also be used to personalize customer experiences and provide tailored recommendations.
- Entertainment: AI and ML are playing an increasingly important role in the entertainment industry, from personalized recommendations on streaming platforms to the creation of realistic special effects in movies and video games. ML algorithms can analyze user preferences, generate creative content, and enhance the overall entertainment experience.
The Future of AI and ML Explained Simply
The future of AI and ML is bright and full of promise. As research continues to advance, we can expect to see even more innovative and and impactful applications in the years to come. Some of the exciting possibilities include:
- Advancements in General AI: Continued research in general AI could lead to the development of machines with human-level intelligence and consciousness, opening up new possibilities in areas such as scientific discovery, healthcare, and space exploration.
- Increased Automation: AI and ML will continue to automate many tasks, freeing up human workers to focus on more creative and strategic endeavors. THis will lead to increased productivity, efficiency, and innovation across various industries.
- Ethical Considerations: As AI and ML become more pervasive, it is crucial to address the ethical implications of these technologies, including issues such as bias, privacy, and job displacement.
- Human-AI Collaboration: The future of AI and ML lies not in replacing humans, but in augmenting human capabilities and enabling us to achieve greater things. By working together, humans and AI can adress some of the most pressing challenges facing our world, from climate change and poverty to disease and inequality.
AI vs ML explained simply: While often used interchangeably, Artificial Intelligence and Machine Learning are distinct but closely related fields that are rapidly transforming our world. AI represents the broader goal of creating intelligent machines, while ML focuses on enabling machines to learn from data. By understanding the key differences and exploring the vast applications of these technologies, we can gain a deeper appreciation for there potential to shape our future. As AI and ML continue to evolve, it is crucial to embrace these technologies responsibly, ensuring that they are used for teh benefit of humanity.
This article has provided a basic overview of AI vs ML explained simply. It is important to remember that these are complex fields with ongoing research and development. As these technologies continue to advance, our understanding of their capabilities and limitations will also evolve.
I hope this article has provided you with a clear and concise understanding of AI vs ML explained simply. If you have any further questions or would like to delve deeper into specific aspects of these technologies, I encourage you to explore additional resources and continue your learning journey